Large language models (LLMs) are exceptionally good at processing requests for information, sifting through vast swaths of data and confidently presenting answers. And while those abilities don’t translate into actual intelligence, there are real similarities between AI and human brains. For example, both use information processing systems that use either biological or artificial neurons to perform computations.
Now, there is a burgeoning area of AI research focused on studying these systems using insights gleaned from human brains. Ultimately, this work could pave the way for more powerful, functional AIs.
Anna Ivanova, 30, is digging into what these models are capable of—and what they aren’t. As assistant professor of psychology at Georgia Institute of Technology, she applies to LLMs some of the same methods that cognitive scientists use to figure out how the human brain works.
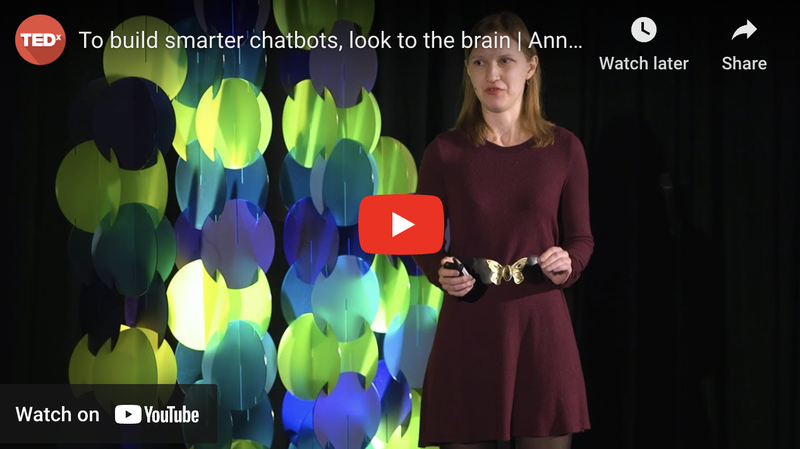
For example, neuroscientists have spent a lot of effort trying to understand how different parts of the brain relate to cognitive abilities. Are individual neurons or regions specialized for a given cognitive function, or are they all multipurpose? How exactly do these components contribute to a system’s behavior?
Ivanova thinks these same questions are key to understanding the internal organization of an artificial neural net and why these models work the way they do. She and her team have studied LLM performance on two essential aspects of language use in humans—formal linguistic competence, which includes knowledge of a language’s rules and patterns, and functional linguistic competence, which involves the cognitive abilities required to understand and use language (like the ability to reason) and social cognition. They did this with prompts, by asking the LLMs, for example: “The meaning of life is…”
While LLMs perform well on formal linguistic competence tasks, they tend to fail many tests involving functional competence. “We're trying to figure out what's going on,” she says. Which, in turn, could help researchers better understand our own brains.
Although it’s important to acknowledge which insights from neuroscience don’t transfer to artificial systems, it’s exciting to be able to use some of the same tools, Ivanova says. She hopes that by understanding how an AI model’s inputs affect the way that system behaves, we will be able to create AI that’s more useful for humans.