Our immune systems are vastly complex networks involving hundreds of proteins that send signals to dozens of cells. Ang Cui, 34, uses machine learning to engineer tools that can make sense of such huge amounts of data. She developed her first machine learning model—an algorithm to study childhood arthritis—as an undergraduate studying computer engineering.
Cui has now applied her computational skills to understand why men’s and women’s immune systems appear to work differently, especially in old age. “Older men are more vulnerable to infections and cancer, whereas older women and women in general are more susceptible to autoimmune diseases,” she says.
Cui developed a tool to analyze genetic mutations in B cells—a type of immune cell that makes antibodies—from healthy volunteers. She analyzed just over 985,000 mutations, a large-scale approach that helped her identify a key molecular pathway important for the development of antibodies and one that seems to differ in older men and women.
Cui, now at Harvard University, has spent 10 years working out the best way to use big data to understand the immune system. Take, for example, cytokines: proteins that direct other immune cells. There are over a hundred of them, each influencing around 18 types of immune cell. Getting a clear picture of all the various possible interactions was generally considered impossible. “Nobody ever really tried to do it,” says Cui.
Cui set out to change that by injecting 86 cytokines into mice and measuring the responses of 17 cell types. She built a computational tool to analyze her data and began compiling information about how each cytokine influences each type of cell. The result is the Immune Dictionary, which Cui describes as being “like a periodic table” for the immune system.
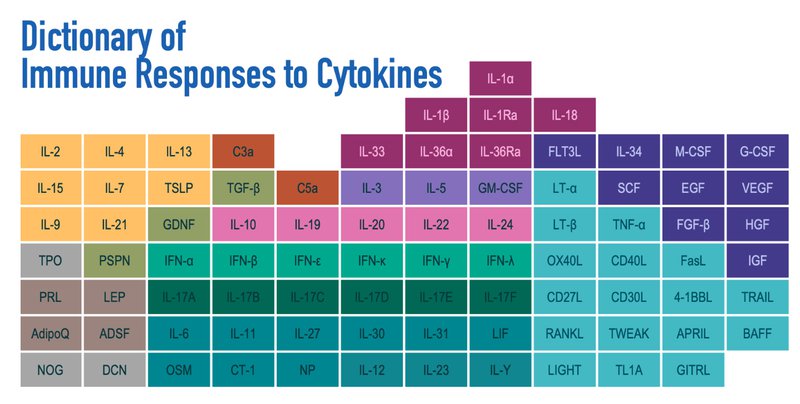
Although it was compiled using data collected from mice, the Immune Dictionary can give researchers insight into how the human immune system works. Cui and her colleagues have created free online software to allow scientists to make use of it. Within days of its launch in December, the web portal “completely crashed” because it was so popular, says Cui—since then, the dictionary has been accessed over 181,000 times.